QUANTITATIVE REASONING EXERCISES USING FUNDAMENTAL STATISTICAL CONCEPTS; STATISTICAL INFERENCE AND HYPOTHESIS TESTING
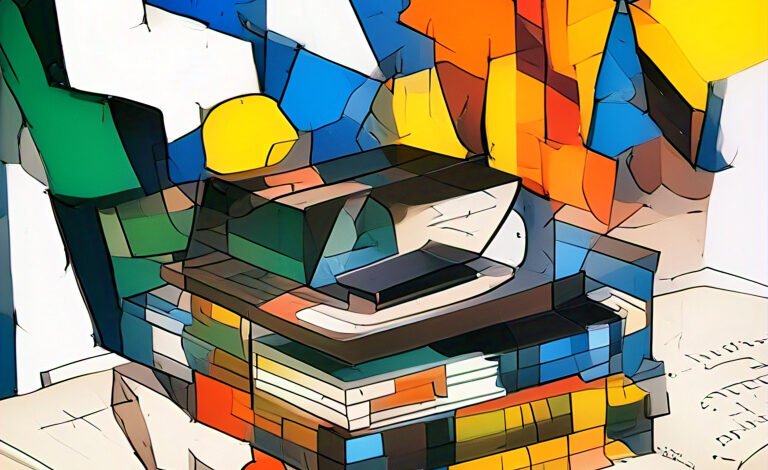
QUANTITATIVE REASONING EXERCISES USING FUNDAMENTAL STATISTICAL CONCEPTS; STATISTICAL INFERENCE AND HYPOTHESIS TESTING
Statistical Inference is described as the process of making conclusions from the result on the basis of chance variation of the data. The two findings of statistical inference include hypothesis testing and confidence interval. Statistical inference is defined as the procedure of reaching decisions about the characteristics of a population by means of random sampling. It also helps us to determine the extent of correlation between dependent variable and independent variable. Statistical inference is based on the fact that in practice we are interested in estimating the uncertainty we have or the sample to sample variation. It allows to obtain a scope of value for the true value of something in the population. The components used for making the statistical inference are:
- Sample Size
- Variability in the sample
- Size of the observed difference
Types of Statistical Inference
There are different forms of statistical inference which are used in making some conclusion for instance; Pearson coefficient of correlation Bi-varaite regression analysis Multivariate regression analysis Anova/T-test Ch-square statistic and contingency table. But, the most important two types of statistical inference that are primarily used are:
- Confidence Interval
- Hypothesis testing
Statistical Inference Procedure
Steps in the Statistical Inference Process:
- Start with a theory: Begin by having an idea or theory about something.
- Create a research hypothesis: Develop a statement that you want to test in your research.
- Identify the variables: Choose the factors or things you will study.
- Identify the target population: Decide who or what your results will apply to.
- Formulate a null hypothesis: Make a prediction that says there is no difference or effect in the population.
- Select a sample: Pick a group from the population (like children) and start the study.
- Run a statistical test: Use a test to see if the results from the sample are different enough from what the null hypothesis predicts. If they are, you can reject the null hypothesis.
Importance of Statistical Inference:
Statistical inference is important because it helps analyze data correctly. It allows researchers to interpret results accurately, which leads to better solutions. By using inferential statistics, we can make predictions about future events in various fields. It helps us estimate a range of values that likely represents the true value in a population. Statistical inference is used in fields such as:
- Business analysis
- Artificial intelligence
- Financial analysis
- Fraud detection
- Machine learning
- Pharmaceutical industry
- Stock market analysis
Statistical Inference Examples
Example: 1
From the Shuffled Pack of 52 Cards, a Card is Drawn. The Trial is Repeated for a Maximum of 400 Times and Different Suits of Cards are Given Below:
Suit | Spade | Clubs | Hearts | Diamond |
No. of times cards drawn | 90 | 100 | 120 | 90 |
What is the probability of getting the following suits, if the card is drawn randomly?Â
- Diamond Card
- Black Card
- Except for spade
Solution: Through statistical inference solution.
Total number of events = 400
i.e. 100 + 90+120+90 = 400
- The probability of winning a diamond card is :
Total number of trials in which diamond card is drawn = 90
Hence, P(diamond card ) = 90/4000
= 0.22
- The probability of winning black card is :
Number of trial in which black card appeared = 100 + 90 = 190
Hence, P(black card) = 190./400 = 0.48
- Except spade
Number of trials other than spade appeared = 90 + 100 + 120 = 310
Hence, P(except spade) = 310/400 = 0.78
Example 2:Â Â 1. A bad containing 2 yellow balls, 3 red balls, and 5 black balls. Only one ball is drawn at random from the bag. What is the probability that the black ball is drawn?
Solution: Through statistical inference solution.
Total number of balls in a bag = 10
i.e 2 + 3+ 5 = 10
Number of black balls= 5
Probability of getting a black balls =Â Number of balck balls/ Total number of balls
= 5/10
= 1/2
Hence, the probability of getting black balls is 1/2.
Example 3:Â Â A card is drawn from a pack of 52 cards. What is the probability that the card drawn is a face card ( Jack, King and Queen only)?
Solution: Total number of cards= 52
Number of a face card in a pack of 52 cards= 12
Probability of receiving a face card = 12/52 = 3/13
Hence, the probability of receiving face card is 3/13
HYPOTHESIS TESTING
Hypothesis testing in statistics is a way to check if an assumption we make about a large group of people or things is likely to be true. It helps us make a smart guess using data. By looking at a small sample from the group, we try to see if the assumption can apply to the entire group.
When we make a guess (hypothesis), it could be right or wrong. It’s our job to find evidence to see if it might be true. To do this, we follow these four simple steps:
- First, we come up with two possible guesses: one is what we think is true, and the other is the opposite. Only one can be correct, and we’ll test both.
- Next, we decide on a statistical method to check the data based on what kind of data we have.
- Then, we analyze the data using the method we chose.
- Finally, we look at the results to decide whether our original guess (called the “null hypothesis”) should be rejected or accepted.
Examples of Hypothesis Testing in Real Life
Here are some examples of hypothesis testing in real life:
- It’s known that the average recovery time after knee surgery is 8 weeks. A doctor thinks that if a patient goes to physical therapy twice a week instead of three times, it will take longer to recover. We can test this assumption by looking at data.
- Saad is a 10-year-old swimmer who usually finishes a 25-yard freestyle race in 16.43 seconds. His dad believes that wearing new goggles will help him swim faster. After timing Saad’s 15 races, the average time came out to 16 seconds. We can test whether the goggles really made a difference.
- A tire company claims that their tires can last 50,000 miles before needing replacement, with a typical difference of 8,000 miles. After testing 28 tires, the average lifespan came out to 46,500 miles with a difference of 9,800 miles. We can test if the company’s claim is accurate.
These examples show that hypothesis testing is practical and used in real-life situations. It’s an important part of research and is applied in many fields.
In inferential statistics, hypothesis testing is a key method. The research hypothesis suggests there is a connection between two factors, while the null hypothesis says there is no such connection. Our job is to figure out if these factors are truly related or not.
Procedure of Hypothesis Testing
To check if a statement is true, we need to follow specific steps for hypothesis testing. After we make the first guess (the initial hypothesis), we rewrite it as two possibilities: the “null hypothesis” and the “alternate hypothesis.” The alternate hypothesis says there is a connection between the things we are looking at (variables), while the null hypothesis says there is no connection.
After writing them as H0(null hypothesis) and Ha(Alternate hypothesis), only one of the statements can be true. For example, taking the hypothesis that, on average, men are taller than women, we write the statements as:
- H0: On average, men are not taller than women.
- Ha: On average, men are taller than women.
Our next aim is to collect sample data, what we call sampling, in a way so that we can test our hypothesis. Your data should come from the concerned population for which you want to make a hypothesis.
What is the p value in hypothesis testing? P-value gives us information about the probability of occurrence of results as extreme as observed results.
You will obtain your p-value after choosing the hypothesis testing method, which will be the guiding factor in rejecting the hypothesis. Usually, the p-value cutoff for rejecting the null hypothesis is 0.05. So anything below that, you will reject the null hypothesis.Â
A low p-value means that the between-group variance is large enough that there is almost no overlapping, and it is unlikely that these came about by chance. A high p-value suggests there is a high within-group variance and low between-group variance, and any difference in the measure is due to chance only.
Types of Hypothesis Testing
There are different kinds of hypothesis tests, and the choice depends on the type of data and the sample size. The method of testing varies according to the data being analyzed. Here’s a summary of common hypothesis testing methods:
- Normality Test: This test checks if a sample follows a normal distribution, where data points cluster around the mean. In a normal distribution, the chance of a value being above or below the mean is equal, forming a bell-shaped curve that is symmetrical around the mean.
- T-test: This test is used for small sample sizes (usually less than 30) from a normally distributed population where the standard deviation is unknown. The T-test helps estimate the confidence intervals for the population mean.
- Chi-Square Test: This test evaluates how well the observed data fits a certain expected distribution. It can also be used to compare population variance with a known or assumed value. Common types of this test include the Chi-Square test of independence and the Chi-Square test of variance.
- ANOVA (Analysis of Variance): ANOVA is used to compare the means of two or more groups or populations. While similar to the T-test or Z-test, ANOVA can compare more than two groups at once. It tests whether there is a significant relationship between an independent variable (X) and a dependent variable (Y).
- Z-test: This test compares the means of two populations when the sample size is large (typically more than 30) and the population variance is known. A Z-test assumes that the population follows a normal distribution, and due to the central limit theorem, larger sample sizes are considered to be normally distributed.